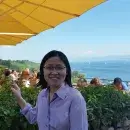
Xiaohong Chen
Xiaohong Chen has made fundamental contributions to econometric methods. Her work on sieve methods for semiparametric and nonparametric models has made the use of flexible functional forms feasible for a wide variety of empirical applications in economics. Much of this work is focused on models with endogenous variables, which are ubiquitous in economic applications, but are difficult to analyze in semiparametric and nonparametric scenarios. Chen also has made important contributions to nonclassical measurement error methods, copula methods in finance, and asset pricing models. Chen's research is characterized by originality, generality, and very high quality.
Xiaohong Chen's current research interests include but not limited to: (1) robust but computationally attractive inference methods for partially identified parametric and semiparametric models; (2) identification, estimation and inference of models combining different macro and micro random variables and various data sources; (3) robust dynamic models with high dimensional conditioning variables that could be flexibly approximated by multi-layer neural networks (i.e., deep learning)
In addition to her regular teaching, advising and research activities, she is also busy with being an editor of Journal of Econometrics (the top field journal in econometrics) and an associate editor of Econometrica (the top-ranked journal in economics as a whole). She is also a chair of the committee supervising China Meetings of the Economic Society (from July 1, 2018 to June 30, 2021).