The Implicit Association Test
Among the general public and behavioral scientists alike, the Implicit Association Test (IAT) is the best known and most widely used tool for demonstrating implicit bias: the unintentional impact of social group information on behavior. More than forty million IATs have been completed at the Project Implicit research website. These public datasets are the most comprehensive documentation of IAT and self-reported bias scores in existence. In this essay, we describe the IAT procedure, summarize key findings using the IAT to document the pervasiveness and correlates of implicit bias, and discuss various ways to interpret IAT scores. We also highlight the most common uses of the IAT. Finally, we discuss unanswered questions and future directions for the IAT specifically, and implicit bias research more generally.
MON____
PAN____
SHE____
Fill in the blanks to complete the words above. What did you come up with? Imagine that before responding to these word stems, you were casually exposed to a list of animal names. Research shows that, in that case, you would be more likely to complete the stems with Monkey, Panda, and Sheep than Monday, Pancake, and Sheet. This residual effect of prior learning can occur even if you are unable to recall the animal word list when asked. This example illustrates implicit memory.1 Although never directly instructed to use previous information, people’s responses indicate a residual effect of what they have learned previously.
In 1995, psychologists Anthony G. Greenwald and Mahzarin R. Banaji introduced the idea of implicit attitudes, arguing that the processes underlying implicit memory effects can also apply in the social world.2 In the same way that traces of experience with word lists can influence word stem completions, traces of experiences can also influence evaluations of social groups—even when we are unable to verbally report on those evaluations. Shortly after Greenwald and Banaji first wrote on implicit attitudes, Greenwald published the Implicit Association Test (IAT) as a measure of performance of these implicit social cognitions, including implicit attitudes (evaluations of groups), implicit self-esteem (attitudes toward oneself), and implicit stereotypes (beliefs about traits that are characteristic of a group).3
In this essay, we describe the IAT procedure, summarize key findings using the IAT, and discuss various ways to interpret IAT scores. We also highlight the most common uses of the IAT. Finally, we discuss unanswered questions and future directions for the IAT specifically, and implicit bias research more generally.
The idea behind the IAT is quite simple: people perform tasks better when a response relies on stronger mental links compared to when a response relies on weaker mental links. Because the IAT is a procedure, not a discrete measure, and researchers vary the features of the task depending on their preferences, there is no single IAT. However, most IATs follow the same general format; let us walk through the age-attitudes version of the task.
Participants in the IAT are tasked with sorting words or pictures into categories as quickly and accurately as possible. There are two key blocks of trials within the IAT in which two categories share the same response (such as a key on a computer keyboard, a square block on a touch device). In the block of trials pictured in Figure 1, if an elderly face appears or positive words appear, you would press the “E” key. If a young-adult face or a negative word appears, you would press the “I” key. You would first complete a set of trials sorting words and pictures in this way. And then the categories switch so the young-adult faces and positive words share the same response key, and older-adult faces and negative words share the same response key, and you would go through the process again with the updated pairings.
All the while, the computer is recording how long it takes for you to make a correct response on each trial. An IAT score reflects the standardized difference in average response time between the two sorting conditions. If someone completes the task faster when young people and positive words share the same response key, and old people and negative words share the same response key—as in the bottom picture in Figure 1—their IAT score would reflect an implicit bias favoring young people over old people. If they complete the task faster when old people and positive words share the same response key and young people and negative words share the same response key—as in the top picture—their IAT score would reflect an implicit bias favoring old people over young people.4
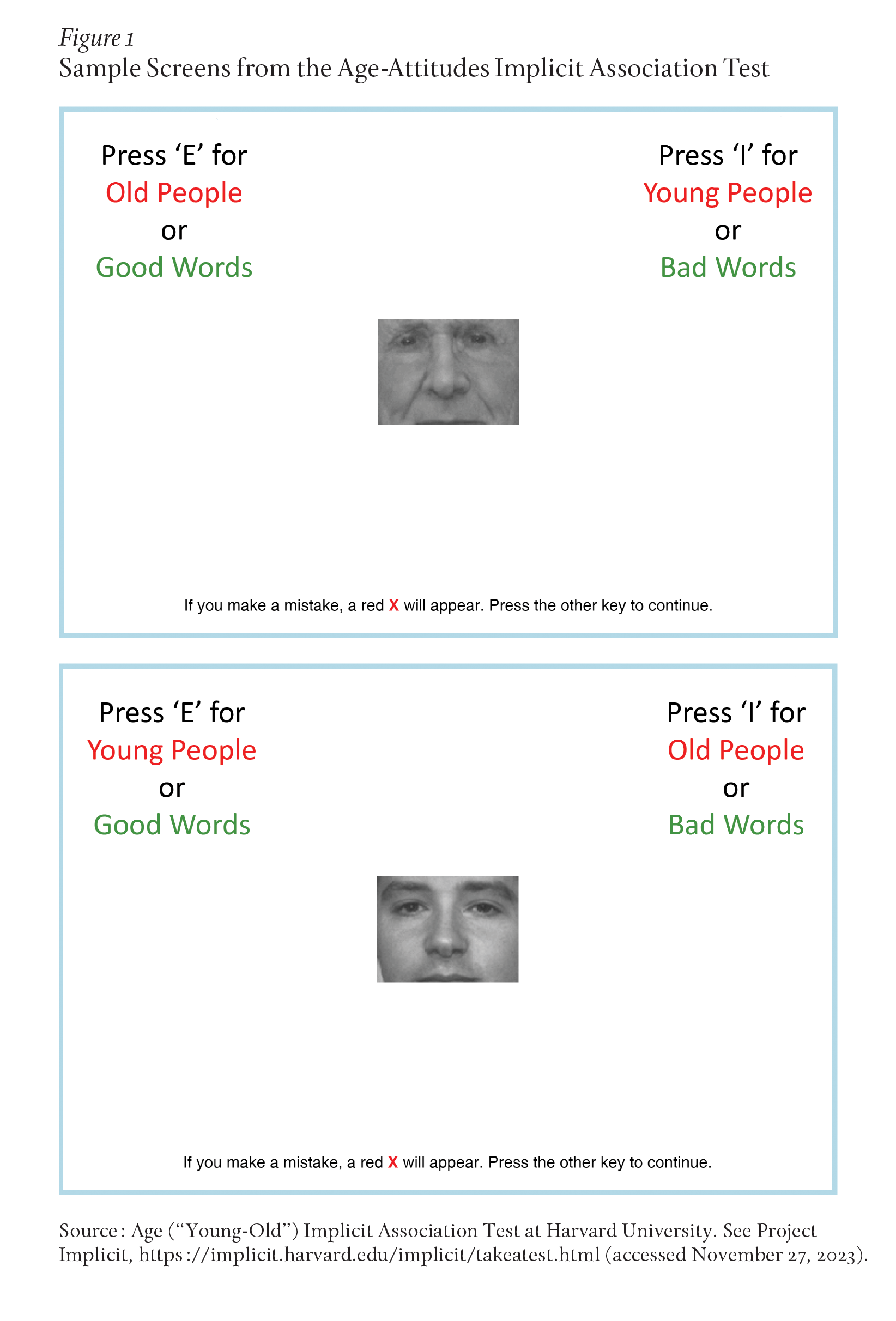
In 2003, Greenwald and Banaji, together with psychologist Brian Nosek, incorporated Project Implicit, a nonprofit organization with a public education mission and an international research collaboration between behavioral scientists interested in implicit social cognition. The core feature of Project Implicit is a demonstration website, set up in the model of an interactive exhibit at a science museum, where visitors can complete an IAT on a topic of their choice. As of late 2023, more than eighty million study sessions have been launched and more than forty million IATs completed at the Project Implicit website—an IAT every twenty-one seconds.5 In addition, there is an uncounted multitude of people who have interacted with the IAT in classroom settings or as part of an educational session at their place of work.
Over the past twenty-five years, we have learned a lot about implicit bias as measured by the IAT. Greenwald and colleagues’ paper introducing the IAT has been cited more than sixteen thousand times since 1998. Across the forty million IATs completed at the Project Implicit website, IAT scores reflect a moderate to strong bias for systematically advantaged groups over systemically disadvantaged or minoritized groups. As seen in Figure 2, there is a clear pattern in favor of straight people (relative to gay people), thin people (relative to fat people), abled people (relative to disabled people), White people (relative to Black people), cisgender people (relative to transgender people), and young people (relative to old people). Notably, people self-report these same biases, but the strength of these biases are considerably weaker.
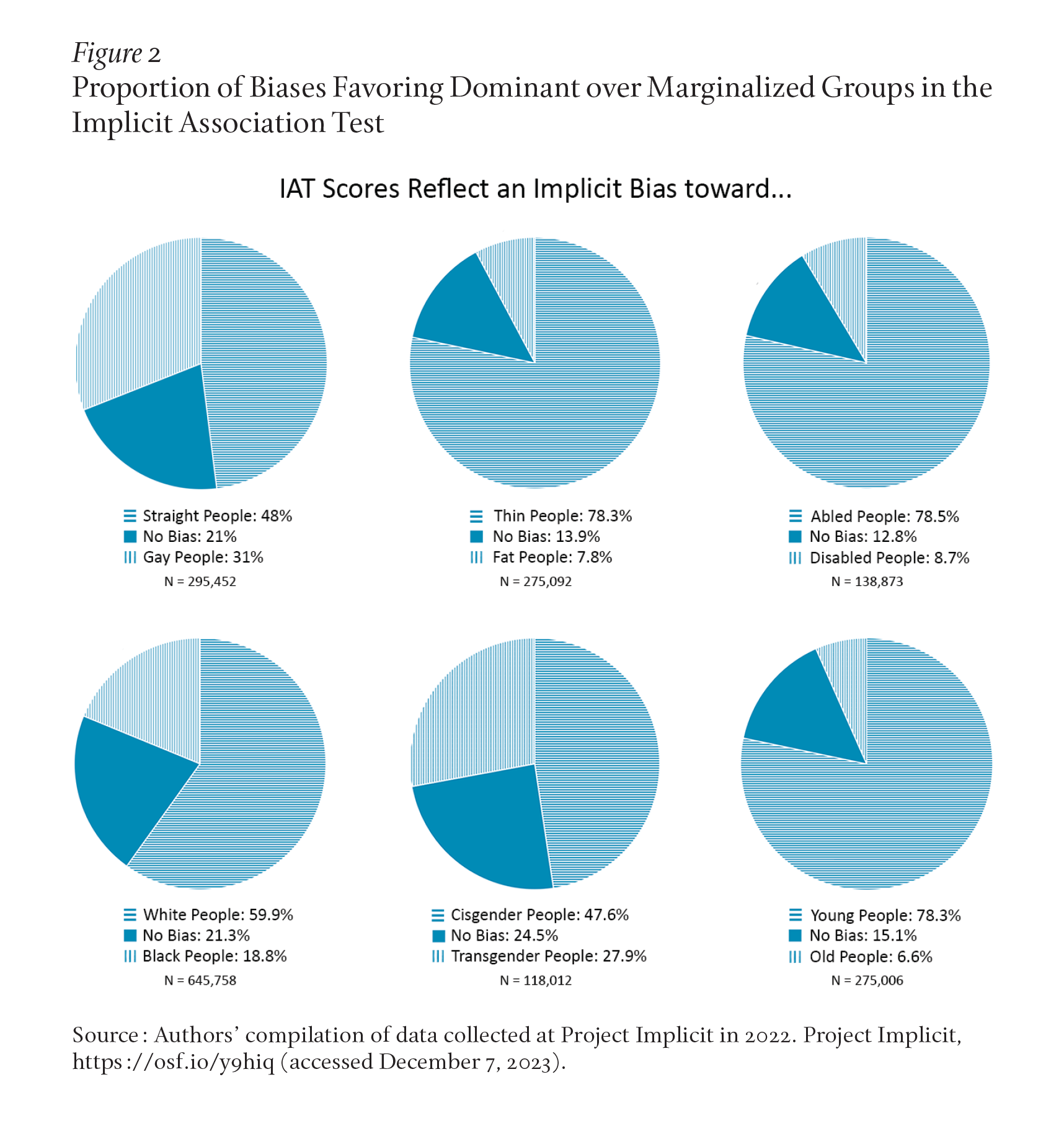
A notable limitation of the IAT, like most other implicit measures, is that it assesses evaluations based on only one clear identity or social group at a time. In real life, of course, people have multiple identities and these identities intersect. In other words, people belong to age and racial and gender groups, and these identities intersect to produce different patterns of experiences, both for the target and perceiver. People’s identities in real life are often also far more ambiguous than the stimuli used in implicit measures of bias.
In addition to the direction and strength of an IAT score (that is, which group it favors and whether we describe it as slight, moderate, or strong), we can also think about the pervasiveness of IAT-measured implicit bias by looking at the percentages of respondents on each task whose IAT score indicates a bias favoring one group over another. For example, approximately 67 percent of visitors to the Project Implicit website have an IAT score indicating some degree of implicit bias toward White people (relative to Black people). And we see similar patterns of IAT scores on tasks indicating an implicit bias toward thin people (relative to fat people), abled people (relative to disabled people), straight people (relative to gay people), young people (relative to old people), and cisgender people (relative to transgender people).
Overall, there are few individual variables that consistently relate to IAT scores. Meta-analytically across all the tasks at the Project Implicit site that are about social groups, we see essentially no relationship between IAT scores and education, religiosity, or age, and we see small relationships between IAT scores and prior IATs completed, political orientation, and gender. There are two factors that correlate fairly substantially with IAT scores. One is self-reported attitudes. People who report having more bias also have more biased performance on the IAT. The other factor that matters consistently across almost every task is relevant group membership.
A much higher percentage of heterosexual participants than gay, lesbian, and bisexual participants have an IAT score that reflects bias in favor of straight people: 62 percent compared to 27 percent. Similarly, a higher percentage of White participants than Black participants have an IAT score reflecting an implicit bias toward White people relative to Black people: 73 percent compared to 41 percent. That said, it is not trivial that 41 percent of Black participants have an IAT score reflecting an implicit bias in favor of White people (Figure 3).
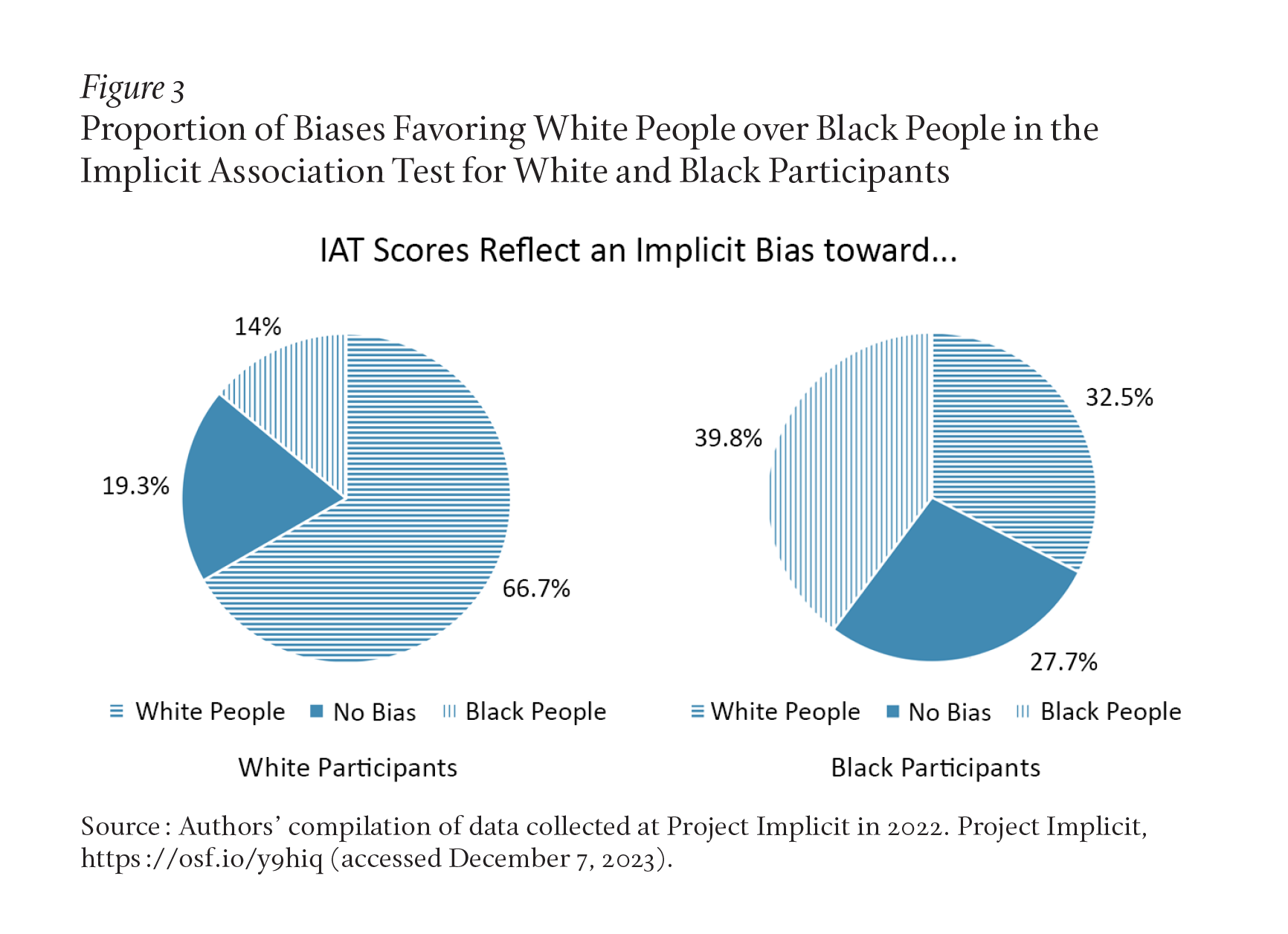
Another opportunity that this accumulated data set of IAT scores affords researchers is the ability to track whether levels of implicit bias have changed over time. Banaji and psychologist Tessa Charlesworth summarized patterns of change among 7.1 million data points collected between 2007 and 2020.6 They found that IAT scores evidencing preferences for young people (relative to old people), abled people (relative to disabled people), and fat people (relative to thin people) have remained fairly stable over time, but preferences for lighter skin (relative to darker skin), White people (relative to Black people), and straight people (relative to gay people) have all decreased in magnitude (that is, shifted toward neutrality over time). This rate of reduction is particularly remarkable for the latter task. Bias favoring straight people (relative to gay people) was reduced by 65 percent across the thirteen-year period sampled. It is also worth noting that these rates of change are happening more quickly for some people than for others. For example, younger people and political liberals showed a larger decrease in implicit anti-gay bias and implicit anti-Black bias than did older people and political conservatives. To be clear, those decreases are evident in all groups, but they are happening faster among some people than others.7
Another approach to looking at the influence of time on IAT scores is to compare average IAT scores in some time frame before and after a particular event. For example, the IAT-measured preference for White people (relative to Black people) in the United States is greater when the economy is worse, and the preference for thin people (relative to fat people) was higher shortly after twenty different highly publicized fat-shaming statements made by celebrities.8 In addition, the bias on the IAT favoring straight people (relative to gay people) decreased at the state level with implementation of same-sex marriage legalization.9 In sum, it is clear that IAT scores change slowly over time and also respond to temporary fluctuations in current events.
When drawing so many conclusions based on one data source, it is important to point out that visitors to the Project Implicit website are certainly not representative of the population from which they are drawn. That said, in terms of sheer numbers, the number of data points in the Project Implicit sample is bigger than the total combined population of eighteen U.S. states. It is certainly the largest database of IAT scores in existence and probably the largest for self-reported biases as well. There is also growing evidence that data from Project Implicit samples perform similarly to those collected from nationally representative samples.10 Thus, because of the scale of IAT data available, it can provide a reasonably good inference about societal-level trends that can complement traditional self-report surveys such as those collected by Gallup or Pew Research Center that rely on random—though generally still not representative—sampling.
You may have noticed that, so far, we have described and discussed IAT scores. The data make clear that IAT scores suggest strong and pervasive biases favoring dominant, societally privileged groups over those that are marginalized and minoritized. But how should we think about what IAT scores are, and what implicit bias is?
One of the central tasks of the behavioral sciences is developing procedures and measures to serve as a proxy for psychological constructs. With traditional self-report measures of psychological constructs, this can be straightforward. For example, the ten-item Rosenberg Self-Esteem Scale asks people the extent to which they agree with items like “On the whole, I am satisfied with myself” and “I have a positive attitude toward myself.”11 This type of instrument is high in face validity; in other words, the measurement procedure makes logical sense as a way to assess the construct of interest. The IAT, however, is not as high in face validity. There is quite a leap between the procedure—sorting words and pictures into categories—and what the test purports to measure—evaluations of social groups. Thus, to demonstrate that the IAT can in fact measure evaluations of social groups, we need to look to other kinds of validity. For example, the IAT relates to other measures of evaluations (convergent validity), it does not relate to measures it should be different from (discriminant validity), and it varies based on one’s own group memberships, as discussed previously, in ways that make sense (known groups validity).12 This could be a lengthy discussion, but in sum, the majority of researchers agree that enough validity evidence has accrued to conclude that the IAT does, in fact, serve as a valid and reliable way to assess individual differences in evaluations of and stereotypes about social groups, though perhaps with a bit more noise than self-report measures.13
But let us return to our original questions in this section: what are IAT scores and what is implicit bias? Even after twenty-five years of research, these are still under vigorous debate, with some arguing that the implicitness construct should be done away with altogether due to its ambiguity and lack of precision, or because it offers little above and beyond self-report measures.14 While we disagree with this conclusion, the value of the implicitness construct is one of the most important questions in this line of research, and it is worth summarizing a few of the different ways that scholars think about implicit bias.15
The earliest and probably still most common idea is that implicit biases reflect some kind of latent mental construct—a hidden force inside of people’s minds—that cannot be directly observed. In this view, implicit biases are something people “have,” as in 60 percent of U.S. participants have an implicit bias favoring cisgender people over transgender people. In their 1995 paper introducing implicit cognition, Greenwald and Banaji defined implicit attitudes as “introspectively unidentified (or inaccurately identified) traces of past experience that mediate responses.”16 The interpretation of this definition (though perhaps not the intention) is that implicit biases are outside of conscious awareness and inaccessible to introspection. The field’s reliance on this definition for more than a decade is likely how unconscious bias and implicit bias came to be used synonymously. In line with this interpretation, the Project Implicit website defined implicit attitudes and stereotypes for many years as those that people are “unwilling or unable to report.”
It has become clear, however, that people do have at least some awareness of their biases, as evidenced by stronger correlations between IAT scores and self-report under particular conditions and by the fact that people are at least somewhat able to predict their IAT scores.17 It is increasingly obvious that defining implicit bias as an evaluation that is entirely outside of conscious awareness would functionally eradicate the construct, as we currently have no measures that can meet the burden of proof of producing effects that are entirely outside of conscious awareness.18
We have argued that if we must distinguish between whether an effect is implicit or explicit bias, (un)consciousness is not the best factor by which to do so because awareness: 1) is complex and multifaceted, 2) is nearly impossible to prove, and 3) ignores the importance of an actor’s intentions.19 Instead, we argue that the key feature of the IAT that distinguishes it from the biases that people self-report is automaticity. Psychologists Agnes Moors and Jan De Houwer conceptualize automaticity as a process that influences task performance (that is, behavior in a way that has one or more of the following features: unintentional, goal-independent, autonomous, unconscious, efficient, and/or fast).20 Of the particular features of automaticity, intentionality (whether or not one has control over the startup of a process) and control (whether or not one can override a process once started) are highly relevant to distinguishing between implicit and explicit bias.21
A vexing problem for the latent mental construct approach to implicit bias is that scores on the IAT and other implicit measures demonstrate group-based preferences that are quite large but are also somewhat unstable. In other words, the same person’s score is likely to differ over time, which is not consistent with the idea of deeply ingrained, overlearned unconscious preferences. In response, recent models propose that intergroup attitudes are better understood as group-level constructs. For example, the prejudice-in-places model posits that places can be characterized as biased to the extent that they create predictable, systematic inequalities through formal (for example, laws) and informal (for example, norms) mechanisms that disadvantage some groups relative to others.22 Variations in these regional inequalities then differentially inform individual-level intergroup attitudes. While the prejudice-in-places model does not distinguish between implicit and explicit intergroup attitudes, the “bias of crowds” model takes a similar approach, but focuses on implicit attitudes. It proposes that implicit attitudes across a group of people reflect rather than cause systemic biases. This perspective also assumes that implicit bias reflects what comes to mind most easily at the time, and that measures like the IAT reflect situations more than people. Biases appear stable to the extent that they reflect systemically biased social structures, but they can fluctuate depending on one’s current context. The interpretation of this approach is that IAT scores are much better measures of biases held by places than biases held within minds.23 Or, less radically, that the biases that exist within minds are critically impacted by physical environments.
Support for geographic, intergroup bias comes primarily through research using publicly available data from Project Implicit that aggregate individual IAT scores at some geographic unit (for example, county-level race bias) and then correlate those scores with another indicator that is also aggregated within the same unit, like racial disparities in school discipline, test scores, and police stops.24 Notably, these county-level differences are not random. History casts a long shadow. For example, IAT scores demonstrating anti-Black bias among White people are higher today in counties and states that were more dependent on the labor of enslaved Black people in 1860, suggesting that historical factors create structural inequalities that are transmitted generationally and that lead to implicit biases favoring White people.25
The idea that something as important as racial bias exists in places more so than in people can be a disorienting idea for many of us born and raised within cultures that predominantly treat places and spaces as neutral and passive while prioritizing the importance of individual actors and their internal states and motivations. In general, when most of us think about a concept like sexism, we think about people (like misogynists). We are unlikely to think about spaces causing people to be sexist. Most researchers have a similar bent. Relatedly, the idea that IAT scores reflect context and history is a radical departure from earlier conceptualizations of implicit bias in two ways, by 1) considering inequality and discrimination as a cause, rather than a consequence, of implicit bias, and 2) implying that countering implicit bias may be accomplished more effectively through changing the environments in which we live rather than changing the individuals who live within those environments.
De Houwer provides a compelling argument that rejects the framing of IAT scores as necessarily reflecting implicit, hidden mental biases that reside inside of minds, and instead conceptualizes performance on measures like the IAT as instances of implicitly biased behavior.26 The IAT provides an example of how a behavior—the ability to categorize words and pictures—can be influenced by social group cues even when people do not have the intention to be influenced by those cues. Biased responses on more real-world kinds of tasks, like hiring behavior or performance evaluation, can evidence implicit bias even without measures like the IAT that are supposed to assess some kind of mediating attitude or belief. There are two key benefits to this approach. First, a functional approach allows researchers to circumvent the perplexing situation of using the same name (“implicit”) for both construct and measure. Second, given that the problem of bias is a behavioral problem, it makes sense to define bias in behavioral terms.
Defining IAT performance as an instance of implicitly biased behavior does not render the results described previously about the pervasiveness of IAT scores favoring privileged groups any less meaningful, nor does it invalidate the idea that performance on the IAT may reflect situations, history, and context more than personal attitudes. Instead, this view positions the IAT as an observable form of bias. This framing requires researchers to explain observable biases rather than engaging in interminable (and potentially intractable) debates about unobservable, theorized mental constructs. For example, it is an observable phenomenon that most participants find it easier to pair bad words with faces of old people than with faces of young people. From there, without mention of underlying processes, we can ask questions such as: Why might they do that? What might that mean? Might some people do that more than others? Can we make people stop doing that?
Before concluding, it is worth discussing the promises and pitfalls of using the IAT as a pre-post measure (testing individuals at different points in time to show change) to test the efficacy of interventions. For example, imagine an organization assesses the biases of its human resources (HR) team using a gender stereotyping IAT, provides its employees with some kind of training program, and then administers the IAT again, finding a reduction in the IAT score. Success, right? Not necessarily. While it may be reasonable and desirable in some situations to examine bias reduction in this way, there are two important caveats to note. First, research shows that IAT scores tend to move toward zero from one test session to the next, without anything in particular happening in between. Thus, it is critical that anyone using the IAT to assess bias reduction includes a control condition to ensure that the intervention has decreased IAT-measured bias more than it would have decreased anyway. Second, when assessing bias reduction using the IAT (or any measure of group-based bias), it is important to clarify that the bias itself is the construct of interest. Returning to the example of the HR team training, we would encourage this team to consider what the training itself was about and then assess that. For example, if the training was about fair interviewing practices, the organization could assess the extent to which HR teams implemented such practices. If the training was about ways to decrease disparities in salary, the organization could assess disparities after a year.
It is difficult to predict what the future holds for the IAT. Citation counts continue to increase year over year, and use of the measure continues to expand into increasingly diverse areas of scholarship. It has been evaluated as rigorously as any psychological measure, and has largely stood up to scrutiny. Further, the concept of “implicit bias” has leapt the walls of the academic journals where it has taken on a life of its own. But ideas ebb and flow, and the way behavioral scientists conceptualize implicit bias has changed dramatically over the last decade, with bias no longer being seen exclusively as a product of individual minds, but instead potentially a product of places. Further, the way that racism and biases exert their power evolves across time, and it is unclear how central implicit forms of bias will be to future versions. We continue to argue about the best ways to define implicit bias in the current time, as evidenced by a recent issue of Psychological Inquiry dedicated to the topic.27 And, as mentioned previously, still others argue that researchers should do away with the term “implicit” altogether.28 But in doing so, we would lose something important: a language to talk about the indisputable fact that, regardless of where they come from, people have ingrained prejudices and stereotypes that influence how they see and interpret the world. In our view, implicit bias is ordinary, it is rooted in culture, and it is pervasive, and we will continue to need measures like the IAT to document and quantify these biases.
Endnotes
- 1Mary S. Weldon, Henry L. Roediger, and Bradford H. Challis, “The Properties of Retrieval Cues Constrain the Picture Superiority Effect,” Memory & Cognition 17 (1) (1989): 95–105; and Daniel L. Schacter, “Implicit Memory: History and Current Status,” Journal of Experimental Psychology: Learning, Memory, and Cognition 13 (3) (1987): 501–518.
- 2Anthony G. Greenwald and Mahzarin R. Banaji, “Implicit Social Cognition: Attitudes, Self-Esteem, and Stereotypes,” Psychological Review 102 (1) (1995): 4–27.
- 3Anthony G. Greenwald, Debbie E. McGhee, and Jordan L. K. Schwartz, “Measuring Individual Differences in Implicit Cognition: The Implicit Association Test,” Journal of Personality and Social Psychology 74 (6) (1998): 1464–1480.
- 4For more details regarding IAT procedure and scoring, see Anthony G. Greenwald, Brian A. Nosek, and Mahzarin R. Banaji, “Understanding and Using the Implicit Association Test: I. An Improved Scoring Algorithm,” Journal of Personality and Social Psychology 85 (2) (2003): 197–216.
- 5For more information about Project Implicit, see Kate A. Ratliff and Colin Tucker Smith, “Lessons from Two Decades with Project Implicit,” in The Cambridge Handbook of Implicit Bias and Racism, ed. Jon A. Krosnick, Tobias H. Stark, and Amanda L. Scott (Cambridge: Cambridge University Press, 2023).
- 6Tessa E. S. Charlesworth and Mahzarin R. Banaji, “Patterns of Implicit and Explicit Stereotypes III: Long-Term Change in Gender Stereotypes,” Social Psychological and Personality Science 13 (1) (2022): 14–26.
- 7Tessa E. S. Charlesworth and Mahzarin R. Banaji, “Patterns of Implicit and Explicit Attitudes: I. Long-Term Change and Stability from 2007 to 2016,” Psychological Science 30 (2) (2019): 174–192; and Charlesworth and Banaji, “Patterns of Implicit and Explicit Stereotypes III.
- 8Emily C. Bianchi, Erika V. Hall, and Sarah Lee, “Reexamining the Link Between Economic Downturns and Racial Antipathy: Evidence that Prejudice Against Blacks Rises During Recessions,” Psychological Science 29 (10) (2018): 1584–1597; and Amanda Ravary, Mark W. Baldwin, and Jennifer A. Bartz, “Shaping the Body Politic: Mass Media Fat-Shaming Affects Implicit Anti-Fat Attitudes,” Personality and Social Psychology Bulletin 45 (11) (2019): 1580–1589.
- 9Eugene K. Ofosu, Michelle K. Chambers, Jacqueline M. Chen, and Eric Hehman, “Same-Sex Marriage Legalization Associated with Reduced Implicit and Explicit Antigay Bias,” Proceedings of the National Academy of Sciences 116 (18) (2019): 8846–8851.
- 10Ibid; and Eric Hehman, Jimmy Calanchini, Jessica K. Flake, and Jordan B. Leitner, “Establishing Construct Validity Evidence for Regional Measures of Explicit and Implicit Racial Bias,” Journal of Experimental Psychology: General 148 (6) (2019): 1022–1040.
- 11Morris Rosenberg, Society and the Adolescent Self-Image (Princeton, N.J.: Princeton University Press, 1965).
- 12Anthony G. Greenwald and Calvin K. Lai, “Implicit Social Cognition,” Annual Review of Psychology 71 (2020): 419–445; and Anthony G. Greenwald, Miguel Brendl, Huajian Cai, et al., “Best Research Practices for Using the Implicit Association Test,” Behavior Research Methods 54 (3) (2022): 1161–1180.
- 13Paul Connor and Ellen R. K. Evers, “The Bias of Individuals (in Crowds): Why Implicit Bias is Probably a Noisily Measured Individual-Level Construct,” Perspectives on Psychological Science 15 (6) (2020): 1329–1345.
- 14Olivier Corneille and Mandy Hütter, “Implicit? What Do You Mean? A Comprehensive Review of the Delusive Implicitness Construct in Attitude Research,” Personality and Social Psychology Review 24 (3) (2020): 212–232; and Ulrich Schimmack, “The Implicit Association Test: A Method in Search of a Construct,” Perspectives on Psychological Science 16 (2) (2021): 396–414. See also Benedek Kurdi, Kate A. Ratliff, and William A. Cunningham, “Can the Implicit Association Test Serve as a Valid Measure of Automatic Cognition? A Response to Schimmack (2021),” Perspectives on Psychological Science 16 (2) (2021): 422–434.
- 15Kate A. Ratliff and Colin Tucker Smith, “Implicit Bias as Automatic Behavior,” Psychological Inquiry 33 (3) (2022): 213–218; and Bertram Gawronski, Alison Ledgerwood, and Paul W. Eastwick, “Reflections on the Difference Between Implicit Bias and Bias on Implicit Measures,” Psychological Inquiry 33 (3) (2022): 219–231.
- 16Greenwald and Banaji, “Implicit Social Cognition,” 5.
- 17Kate A. Ranganath, Colin Tucker Smith, and Brian A. Nosek, “Distinguishing Automatic and Controlled Components of Attitudes from Direct and Indirect Measurement Method,” Journal of Experimental Social Psychology 44 (11) (2008): 386–396; Adam Hahn, Charles M. Judd, Holen K. Hirsh, and Irene V. Blair, “Awareness of Implicit Attitudes,” Journal of Experimental Psychology: General 143 (3) (2014): 1369–1392; and Adam Morris and Benedek Kurdi, “Awareness of Implicit Attitudes: Large-Scale Investigations of Mechanism and Scope,” Journal of Experimental Psychology: General 152 (12) (2023): 3311–3343.
- 18To be clear, the idea that people harbor internalized prejudices and stereotypes that influence how they see and interpret the world is not dependent on the existence or fitness of any particular measure. Put another way, implicit bias will still exist without the IAT.
- 19Ratliff and Smith, “Implicit Bias”; John A. Bargh, “The Four Horsemen of Automaticity: Awareness, Intention, Efficiency, and Control,” in Handbook of Social Cognition: Basic Processes; Applications, ed. Robert S. Wyer Jr. and Thomas K. Srull (Mahwah, N.J.: Lawrence Erlbaum Associates, Inc., 1994), 1–40; Adam Hahn and Alexandra Goedderz, “Trait-Unconsciousness, State-Unconsciousness, Preconsciousness, and Social Miscalibration in the Context of Implicit Evaluation,” Social Cognition 38 (S) (2020): 115–134; and Richard E. Nisbett and Timothy D. Wilson, “Telling More Than We Can Know: Verbal Reports on Mental Processes,” Psychological Review 84 (3) (1977): 231–259.
- 20Agnes Moors and Jan De Houwer, “Automaticity: A Theoretical and Conceptual Analysis,” Psychological Bulletin 132 (2) (2006): 297–326; and Jan De Houwer and Agnes Moors, “How to Define and Examine the Implicitness of Implicit Measures,” in Implicit Measures and Attitudes, ed. Bernd Wittenbrink and Norbert Schwarz (New York: The Guilford Press, 2007), 179–194.
- 21Bargh, “The Four Horsemen of Automaticity.”
- 22Mary C. Murphy, Kathryn M. Kroeper, and Elise M. Ozier, “Prejudiced Places: How Contexts Shape Inequality and How Policy Can Change Them,” Policy Insights from the Behavioral and Brain Sciences 5 (1) (2018): 66–74.
- 23B. Keith Payne, Heidi A. Vuletich, and Kristjen B. Lundberg, “The Bias of Crowds: How Implicit Bias Bridges Personal and Systemic Prejudice,” Psychological Inquiry 28 (4) (2017): 233–248; and Manuel J. Galvan and B. Keith Payne, “Implicit Bias as a Cognitive Manifestation of Systemic Racism,” Dædalus 153 (1) (Winter 2024): 106–122.
- 24Travis Riddle and Stacey Sinclair, “Racial Disparities in School-Based Disciplinary Actions Are Associated with County-Level Rates of Racial Bias,” Proceedings of the National Academy of Sciences 116 (17) (2019): 8255–8260; Mark J. Chin, David M. Quinn, Tasminda K. Dhaliwal, et al., “Bias in the Air: A Nationwide Exploration of Teachers’ Implicit Racial Attitudes, Aggregate Bias, and Student Outcomes,” Educational Researcher 49 (8) (2020): 566–578; and Marleen Stelter, Iniobong Essien, Carsten Sander, and Juliane Degner, “Racial Bias in Police Traffic Stops: White Residents’ County-Level Prejudice and Stereotypes Are Related to Disproportionate Stopping of Black Drivers,” Psychological Science 33 (4) (2022): 483–496.
- 25B. Keith Payne, Heidi A. Vuletich, and Jazmin L. Brown-Iannuzzi, “Historical Roots of Implicit Bias in Slavery,” Proceedings of the National Academy of Sciences 116 (24) (2019): 11693–11698.
- 26Jan De Houwer, “Implicit Bias Is Behavior: A Functional-Cognitive Perspective on Implicit Bias,” Perspectives on Psychological Science 14 (5) (2019): 835–840.
- 27Bertram Gawronski, Alison Ledgerwood, and Paul W. Eastwick, “Implicit Bias ≠ Bias on Implicit Measures,” Psychological Inquiry 33 (3) (2022): 139–155.
- 28For comparison, see Corneille and Hütter, “Implicit? What Do You Mean?”; and Greenwald and Lai, “Implicit Social Cognition.”