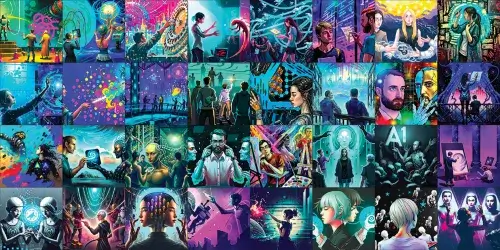
“From So Simple a Beginning”: Species of Artificial Intelligence
Artificial intelligence has a decades-long history that exhibits alternating enthusiasm and disillusionment for the field’s scientific insights, technical accomplishments, and socioeconomic impact. Recent achievements have seen renewed claims for the transformative and disruptive effects of AI. Reviewing the history and current state of the art reveals a broad repertoire of methods and techniques developed by AI researchers. In particular, modern machine learning methods have enabled a series of AI systems to achieve superhuman performance. The exponential increases in computing power, open-source software, available data, and embedded services have been crucial to this success. At the same time, there is growing unease around whether the behavior of these systems can be rendered transparent, explainable, unbiased, and accountable. One consequence of recent AI accomplishments is a renaissance of interest around the ethics of such systems. More generally, our AI systems remain singular task-achieving architectures, often termed narrow AI. I will argue that artificial general intelligence–able to range across widely differing tasks and contexts–is unlikely to be developed, or emerge, any time soon.
If We Succeed
Since its inception, AI has operated within a standard model whereby systems are designed to optimize a fixed, known objective. This model has been increasingly successful. I briefly summarize the state of the art and its likely evolution over the next decade. Substantial breakthroughs leading to general-purpose AI are much harder to predict, but they will have an enormous impact on society. At the same time, the standard model will become progressively untenable in real-world applications because of the difficulty of specifying objectives completely and correctly. I propose a new model for AI development in which the machine’s uncertainty about the true objective leads to qualitatively new modes of behavior that are more robust, controllable, and deferential.
A Golden Decade of Deep Learning: Computing Systems & Applications
The past decade has seen tremendous progress in the field of artificial intelligence thanks to the resurgence of neural networks through deep learning. This has helped improve the ability for computers to see, hear, and understand the world around them, leading to dramatic advances in the application of AI to many fields of science and other areas of human endeavor. In this essay, I examine the reasons for this progress, including the confluence of progress in computing hardware designed to accelerate machine learning and the emergence of open-source software frameworks to dramatically expand the set of people who can use machine learning effectively. I also present a broad overview of some of the areas in which machine learning has been applied over the past decade. Finally, I sketch out some likely directions from which further progress in artificial intelligence will come.
I Do Not Think It Means What You Think It Means: Artificial Intelligence, Cognitive Work & Scale
Over the past decade, AI technologies have advanced by leaps and bounds. Progress has been so fast, voluminous, and varied that it can be a challenge even for experts to make sense of it all. In this essay, I propose a framework for thinking about AI systems, specifically the idea that they are ultimately tools developed by humans to help other humans perform an increasing breadth of their cognitive work. Our AI systems for assisting us with our cognitive work have become more capable and general over the past few years. This is in part due to a confluence of novel AI algorithms and the availability of massive amounts of data and compute. From this, researchers and engineers have been able to construct large, general models that serve as flexible and powerful building blocks that can be composed with other software to drive breakthroughs in the natural and physical sciences, to solve hard optimization and strategy problems, to perform perception tasks, and even to assist with complex cognitive tasks like coding.
Searching for Computer Vision North Stars
Computer vision is one of the most fundamental areas of artificial intelligence research. It has contributed to the tremendous progress in the recent deep learning revolution in AI. In this essay, we provide a perspective of the recent evolution of object recognition in computer vision, a flagship research topic that led to the breakthrough data set of ImageNet and its ensuing algorithm developments. We argue that much of this progress is rooted in the pursuit of research “north stars,” wherein researchers focus on critical problems of a scientific discipline that can galvanize major efforts and groundbreaking progress. Following the success of ImageNet and object recognition, we observe a number of exciting areas of research and a growing list of north star problems to tackle. This essay recounts the brief history of ImageNet, its related work, and the follow-up progress. The goal is to inspire more north star work to advance the field, and AI at large.
The Machines from Our Future
While the last sixty years have defined the field of industrial robots and empowered hard-bodied robots to execute complex assembly tasks in constrained industrial settings, the next sixty years will usher in our time with pervasive robots that come in a diversity of forms and materials and help people with physical tasks. The past sixty years have mostly been inspired by the human form, but the form diversity of the animal kingdom has broader potential. With the development of soft materials, machines and materials are coming closer together: machines are becoming compliant and fluid-like materials, and materials are becoming more intelligent. This progression raises the question: what will be the machines from our future?
Multi-Agent Systems: Technical & Ethical Challenges of Functioning in a Mixed Group
In today’s highly interconnected, open-networked computing world, artificial intelligence computer agents increasingly interact in groups with each other and with people both virtually and in the physical world. AI’s current core challenges concern determining ways to build AI systems that function effectively and safely for people and the societies in which they live. To incorporate reasoning about people, research in multi-agent systems has engendered paradigmatic shifts in computer-agent design, models, and methods, as well as the development of new representations of information about agents and their environments. These changes have raised technical as well as ethical and societal challenges. This essay describes technical advances in computer-agent representations, decision-making, reasoning, and learning methods and highlights some paramount ethical challenges.
Human Language Understanding & Reasoning
The last decade has yielded dramatic and quite surprising breakthroughs in natural language processing through the use of simple artificial neural network computations, replicated on a very large scale and trained over exceedingly large amounts of data. The resulting pretrained language models, such as BERT and GPT-3, have provided a powerful universal language understanding and generation base, which can easily be adapted to many understanding, writing, and reasoning tasks. These models show the first inklings of a more general form of artificial intelligence, which may lead to powerful foundation models in domains of sensory experience beyond just language.
The Curious Case of Commonsense Intelligence
Commonsense intelligence is a long-standing puzzle in AI. Despite considerable advances in deep learning, AI continues to be narrow and brittle due to its lack of common sense. Why is common sense so trivial for humans but so hard for machines? In this essay, I map the twists and turns in recent research adventures toward commonsense AI. As we will see, the latest advances on common sense are riddled with new, potentially counterintuitive perspectives and questions. In particular, I discuss the significance of language for modeling intuitive reasoning, the fundamental limitations of logic formalisms despite their intellectual appeal, the case for on-the-fly generative reasoning through language, the continuum between knowledge and reasoning, and the blend between symbolic and neural knowledge representations.
Language & Coding Creativity
Machines are gaining understanding of language at a very rapid pace. This achievement has given rise to a host of creative and business applications using natural language processing (NLP) engines, such as OpenAI’s GPT-3. NLP applications do not simply change commerce and literature. They raise new questions about how human beings relate to machines and how that symbiosis of communication will evolve as the future rushes toward us.
Non-Human Words: On GPT-3 as a Philosophical Laboratory
In this essay, I investigate the effect of OpenAI’s GPT-3 on the modern concept of the human (as alone capable of reason and language) and of machines (as devoid of reason and language). I show how GPT-3 and other transformer-based language models give rise to a new, structuralist concept of language, implicit in which is a new understanding of human and machine that unfolds far beyond the reach of the categories we have inherited from the past. I try to make compelling the argument that AI companies like OpenAI, Google, Facebook, or Microsoft effectively are philosophical laboratories (insofar as they disrupt the old concepts/ontologies we live by) and I ask what it would mean to build AI products from the perspective of the philosophical disruptions they provoke: can we liberate AI from the concept of the human we inherited from the past?
Do Large Language Models Understand Us?
Large language models (LLMs) represent a major advance in artificial intelligence and, in particular, toward the goal of human-like artificial general intelligence. It is sometimes claimed, though, that machine learning is “just statistics,” hence that, in this grander ambition, progress in AI is illusory. Here I take the contrary view that LLMs have a great deal to teach us about the nature of language, understanding, intelligence, sociality, and personhood. Specifically: statistics do amount to understanding, in any falsifiable sense. Furthermore, much of what we consider intelligence is inherently dialogic, hence social; it requires a theory of mind. Complex sequence learning and social interaction may be a sufficient basis for general intelligence, including theory of mind and consciousness. Since the interior state of another being can only be understood through interaction, no objective answer is possible to the question of when an “it” becomes a “who,” but for many people, neural nets running on computers are likely to cross this threshold in the very near future.
Signs Taken for Wonders: AI, Art & the Matter of Race
AI shares with earlier socially transformative technologies a reliance on limiting models of the “human” that embed racialized metrics for human achievement, expression, and progress. Many of these fundamental mindsets about what constitutes humanity have become institutionally codified, continuing to mushroom in design practices and research development of devices, applications, and platforms despite the best efforts of many well-intentioned technologists, scholars, policy-makers, and industries. This essay argues why and how AI needs to be much more deeply integrated with the humanities and arts in order to contribute to human flourishing, particularly with regard to social justice. Informed by decolonial, disability, and gender critical frameworks, some AI artist-technologists of color challenge commercial imperatives of “personalization” and “frictionlessness,” representing race, ethnicity, and gender not as normative self-evident categories nor monetized data points, but as dynamic social processes always indexing political tensions and interests.
Toward a Theory of Justice for Artificial Intelligence
This essay explores the relationship between artificial intelligence and principles of distributive justice. Drawing upon the political philosophy of John Rawls, it holds that the basic structure of society should be understood as a composite of sociotechnical systems, and that the operation of these systems is increasingly shaped and influenced by AI. Consequently, egalitarian norms of justice apply to the technology when it is deployed in these contexts. These norms entail that the relevant AI systems must meet a certain standard of public justification, support citizens’ rights, and promote substantively fair outcomes, something that requires particular attention to the impact they have on the worst-off members of society.
Artificial Intelligence, Humanistic Ethics
Ethics is concerned with what it is to live a flourishing life and what it is we morally owe to others. The optimizing mindset prevalent among computer scientists and economists, among other powerful actors, has led to an approach focused on maximizing the fulfilment of human preferences, an approach that has acquired considerable influence in the ethics of AI. But this preference-based utilitarianism is open to serious objections. This essay sketches an alternative, “humanistic” ethics for AI that is sensitive to aspects of human engagement with the ethical often missed by the dominant approach. Three elements of this humanistic approach are outlined: its commitment to a plurality of values, its stress on the importance of the procedures we adopt, not just the outcomes they yield, and the centrality it accords to individual and collective participation in our understanding of human well-being and morality. The essay concludes with thoughts on how the prospect of artificial general intelligence bears on this humanistic outlook.
Automation, Augmentation, Value Creation & the Distribution of Income & Wealth
Digital technologies are transforming the economy and society. The dimensionality and scope of the impacts are bewildering and too numerous to cover in a single essay. But of all the concerns around digital technology (and there are many), perhaps none has attracted more attention, and generated deeper anxiety, than the impact of various types of automation on work and on the structure of the economy. I focus on the ways in which the digitization of virtually all data, information, and content is transforming economies. And more specifically, I look at the impacts of automation, augmentation, AI, machine learning, and advanced robotics on economic transformations, on work, and on the distribution of income and wealth.
Automation, AI & Work
We characterize artificial intelligence as “routine-biased technological change on steroids,” adding intelligence to automation tools that substitute for humans in physical tasks and substituting for humans in routine and increasingly nonroutine cognitive tasks. We predict how AI will displace humans from existing tasks while increasing demand for humans in new tasks in both manufacturing and services. We also examine the effects of AI-enabled digital platforms on labor. Our conjecture is that AI will continue, even intensify, automation’s adverse effects on labor, including the polarization of employment, stagnant wage growth for middle and low-skill workers, growing inequality, and a lack of good jobs. Though there likely will be enough jobs to keep pace with the slow growth of the labor supply in the advanced economies, we are skeptical that AI and ongoing automation will support the creation of enough good jobs. We doubt that the anticipated productivity and growth benefits of AI will be widely shared, predicting instead that they will fuel more inequality. Yet we are optimistic that interventions can mitigate or offset AI’s adverse effects on labor. Ultimately, how the benefits of intelligent automation tools are realized and shared depends not simply on their technological design but on the design of intelligent policies.
The Turing Trap: The Promise & Peril of Human-Like Artificial Intelligence
In 1950, Alan Turing proposed a test of whether a machine was intelligent: could a machine imitate a human so well that its answers to questions were indistinguishable from a human’s? Ever since, creating intelligence that matches human intelligence has implicitly or explicitly been the goal of thousands of researchers, engineers, and entrepreneurs. The benefits of human-like artificial intelligence (HLAI) include soaring productivity, increased leisure, and perhaps most profoundly a better understanding of our own minds. But not all types of AI are human-like–in fact, many of the most powerful systems are very different from humans–and an excessive focus on developing and deploying HLAI can lead us into a trap. As machines become better substitutes for human labor, workers lose economic and political bargaining power and become increasingly dependent on those who control the technology. In contrast, when AI is focused on augmenting humans rather than mimicking them, humans retain the power to insist on a share of the value created. What is more, augmentation creates new capabilities and new products and services, ultimately generating far more value than merely human-like AI. While both types of AI can be enormously beneficial, there are currently excess incentives for automation rather than augmentation among technologists, business executives, and policy-makers.
AI, Great Power Competition & National Security
Breakthroughs in AI are accelerating global commercial competition and transforming the international security environment. The reach and influence of foreign-based network platforms present risks to American society and require us to confront questions about their origin and purpose. Meanwhile, AI technologies are enhancing several existing national security threats, and will change the way states try to gain leverage against adversaries and exercise coercion and influence in other societies. The open nature of free and democratic societies, combined with their increasing reliance on poorly secured digital networks, makes them especially vulnerable. In the military realm, AI holds the prospect of augmenting cyber, conventional, and nuclear capabilities in ways that make security relationships among rivals more challenging to predict and maintain, and conflicts more difficult to limit. Even as they compete, rivals should explore limits on AI capabilities. The AI ecosystems of the principal global competitors, the United States and China, remain intertwined, and a calibration of the bilateral technology relationship requires both selective decoupling and continued collaboration in areas of mutual interest. These changes require a comprehensive national strategy for the next decade that preserves global leadership advantages for America’s economy and security.
The Moral Dimension of AI-Assisted Decision-Making: Some Practical Perspectives from the Front Lines
This essay takes an engineering approach to ensuring that the deployment of artificial intelligence does not confound ethical principles, even in sensitive applications like national security. There are design techniques in all three parts of the AI architecture–algorithms, data sets, and applications–that can be used to incorporate important moral considerations. The newness and complexity of AI cannot therefore serve as an excuse for immoral outcomes of deployment by companies or governments.
Distrust of Artificial Intelligence: Sources & Responses from Computer Science & Law
Social distrust of AI stems in part from incomplete and faulty data sources, inappropriate redeployment of data, and frequently exposed errors that reflect and amplify existing social cleavages and failures, such as racial and gender biases. Other sources of distrust include the lack of “ground truth” against which to measure the results of learned algorithms, divergence of interests between those affected and those designing the tools, invasion of individual privacy, and the inapplicability of measures such as transparency and participation that build trust in other institutions. Needed steps to increase trust in AI systems include involvement of broader and diverse stakeholders in decisions around selection of uses, data, and predictors; investment in methods of recourse for errors and bias commensurate with the risks of errors and bias; and regulation prompting competition for trust.
Democracy & Distrust in an Era of Artificial Intelligence
Our legal system has historically operated under the general view that courts should defer to the legislature. There is one significant exception to this view: cases in which it appears that the political process has failed to recognize the rights or interests of minorities. This basic approach provides much of the foundational justifications for the role of judicial review in protecting minorities from discrimination by the legislature. Today, the rise of AI decision-making poses a similar challenge to democracy’s basic framework. As I argue in this essay, the rise of three trends–privatization, prediction, and automation in AI–have combined to pose similar risks to minorities. In this essay, I outline what a theory of judicial review would look like in an era of artificial intelligence, analyzing both the limitations and the possibilities of judicial review of AI. Here, I draw on cases in which AI decision-making has been challenged in courts, to show how concepts of due process and equal protection can be recuperated in a modern AI era, and even integrated into AI, to provide for better oversight and accountability.
Artificially Intelligent Regulation
This essay maps the potential, and risks, of artificially intelligent regulation: regulatory arrangements that use a complex computational algorithm or another artificial agent either to define a legal norm or to guide its implementation. The ubiquity of AI systems in modern organizations all but guarantees that regulators or the parties they regulate will make use of learning algorithms or novel techniques to analyze data in the process of defining, implementing, or complying with regulatory requirements. We offer an account of the possible benefits and harms of artificially intelligent regulation. Its mix of costs and rewards, we show, depend primarily on whether AI is deployed in ways aimed merely at shoring up existing hierarchies, or whether AI systems are embedded in and around legal frameworks carefully structured and evaluated to better our lives, environment, and future.
Socializing Data
Will the proliferation of data enable AI to deliver progress? An ever-growing swath of life is available as digitally captured and stored data records. Effective government, business management, and even personal life are increasingly suggested to be a matter of using AI to interpret and act on the data. This optimism should be tempered with caution. Data cannot capture much of the richness of life, and while AI has great potential for beneficial uses, its delivery of progress in any human sense will depend on not using all the data that can be collected. Moreover, the more digital technology rewires society, creating opportunities for the use of big data and AI, the greater the need for trust and human deliberation.
Rethinking AI for Good Governance
This essay examines what AI can do for government, specifically through three generic tools at the heart of governance: detection, prediction, and data-driven decision-making. Public sector functions, such as resource allocation and the protection of rights, are more normatively loaded than those of firms, and AI poses greater ethical challenges than earlier generations of digital technology, threatening transparency, fairness, and accountability. The essay discusses how AI might be developed specifically for government, with a public digital ethos to protect these values. Three moves that could maximize the transformative possibilities for a distinctively public sector AI are the development of government capacity to foster innovation through AI; the building of integrated and generalized models for policy-making; and the detection and tackling of structural inequalities. Combined, these developments could offer a model of data-intensive government that is more efficient, ethical, fair, prescient, and resilient than ever before in administrative history.